How Generative AI Can Augment Human Creativity
Introduction
Creativity is one of the most distinctive and valuable traits of human beings. It is the ability to produce original and useful ideas, products, or solutions that are not obvious or predetermined. Creativity is often associated with artistic expression, innovation, and problem-solving. However, creativity is not limited to these domains. It is a universal skill that can be applied to any field or activity.
In the ever-evolving landscape of technology, the emergence of generative artificial intelligence (AI) has ignited a captivating conversation about its transformative potential in the realm of creativity. Generative AI refers to a subset of artificial intelligence that uses neural networks to generate content autonomously. One of the most notable examples is GPT-3, a language model capable of producing human-like text. Generative AI can create content across various domains, including text, art, music, video, and more.
The fusion of generative AI and creativity represents a dynamic synergy that is poised to revolutionize the way we approach artistic expression, innovation, and problem-solving. In this article, we will explore how generative AI can augment human creativity, highlighting some of the fascinating examples and applications of this technology in various fields. We will also discuss some of the challenges and opportunities that generative AI poses for the future of creativity.
What is generative AI and how does it differ from other forms of artificial intelligence?
Artificial intelligence is a broad term that encompasses various technologies that enable machines to perform tasks that normally require human intelligence, such as reasoning, learning, decision making, perception, and natural language processing. Artificial intelligence can be classified into two main categories: analytical and generative.
Analytical AI is the most common and familiar form of artificial intelligence. It involves using algorithms to analyze data and provide insights, predictions, or recommendations based on predefined rules or objectives. Examples of analytical AI include search engines, voice assistants, facial recognition systems, spam filters, recommender systems, and self-driving cars.
Generative AI is a relatively new and emerging form of artificial intelligence. It involves using algorithms to generate data or content that is not based on predefined rules or objectives, but rather on learning from existing data or content. Generative AI uses neural networks, which are computational models inspired by the structure and function of biological brains. Neural networks consist of layers of interconnected nodes that process information and learn from data through feedback loops.
One of the most powerful types of neural networks used for generative AI is called Generative Adversarial Networks (GANs). GANs consist of two competing neural networks: a generator and a discriminator. The generator tries to create realistic data or content that mimics the distribution of the original data or content. The discriminator tries to distinguish between the real data or content and the fake data or content generated by the generator. The generator and the discriminator learn from each other through an adversarial process until they reach an equilibrium where the generator produces data or content that is indistinguishable from the real data or content.
Generative AI differs from analytical AI in several ways. First, generative AI does not require a predefined goal or objective. It can create data or content without any specific purpose or direction. Second, generative AI does not rely on explicit rules or logic. It can create data or content that is novel, surprising, or unexpected. Third, generative AI does not produce a single or optimal output. It can create multiple or diverse outputs that vary in quality, style, or complexity.
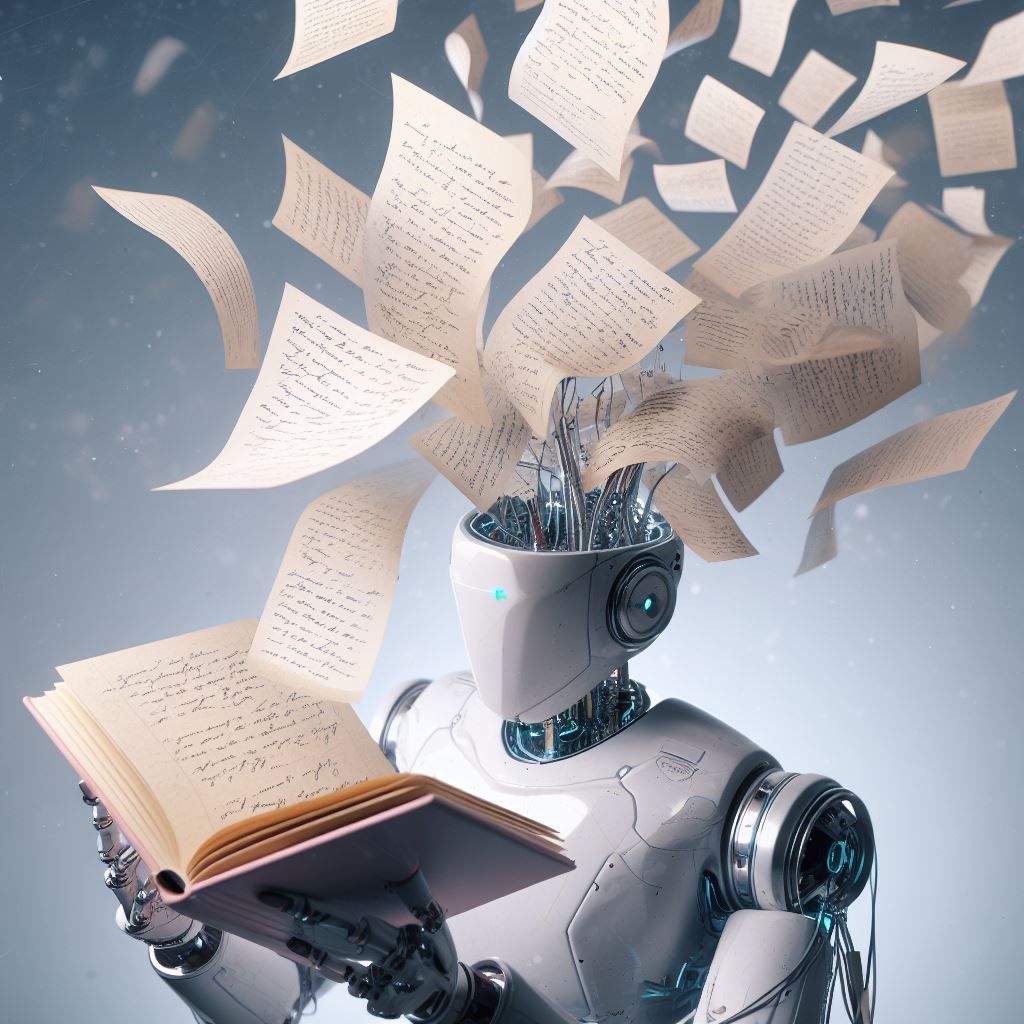
How can generative AI enhance human creativity?
Creativity is often defined as the ability to produce original and useful ideas, products, or solutions that are not obvious or predetermined. Creativity involves two main cognitive processes: divergent thinking and convergent thinking. Divergent thinking is the ability to generate many different ideas or alternatives from a given problem or situation. Convergent thinking is the ability to evaluate, select, and refine the best idea or solution from the generated alternatives.
Generative AI can enhance human creativity by augmenting both divergent and convergent thinking processes. Generative AI can help humans explore new possibilities and generate novel ideas that may not have occurred to them otherwise. Generative AI can also help humans overcome creative blocks and find new sources of inspiration that may stimulate their imagination. Generative AI can also help humans solve complex problems and optimize solutions that may be beyond their reach or expertise. Generative AI can also help humans collaborate with other humans and machines in creative processes, facilitating interactive and co-creative experiences.
Generative AI can augment human creativity in various ways, depending on the domain, context, and purpose of the creative task. In some cases, generative AI can act as a creative tool that humans can use to enhance their own creativity. In other cases, generative AI can act as a creative partner that humans can interact with and learn from. In some cases, generative AI can even act as a creative agent that humans can delegate or outsource some aspects of the creative task to.
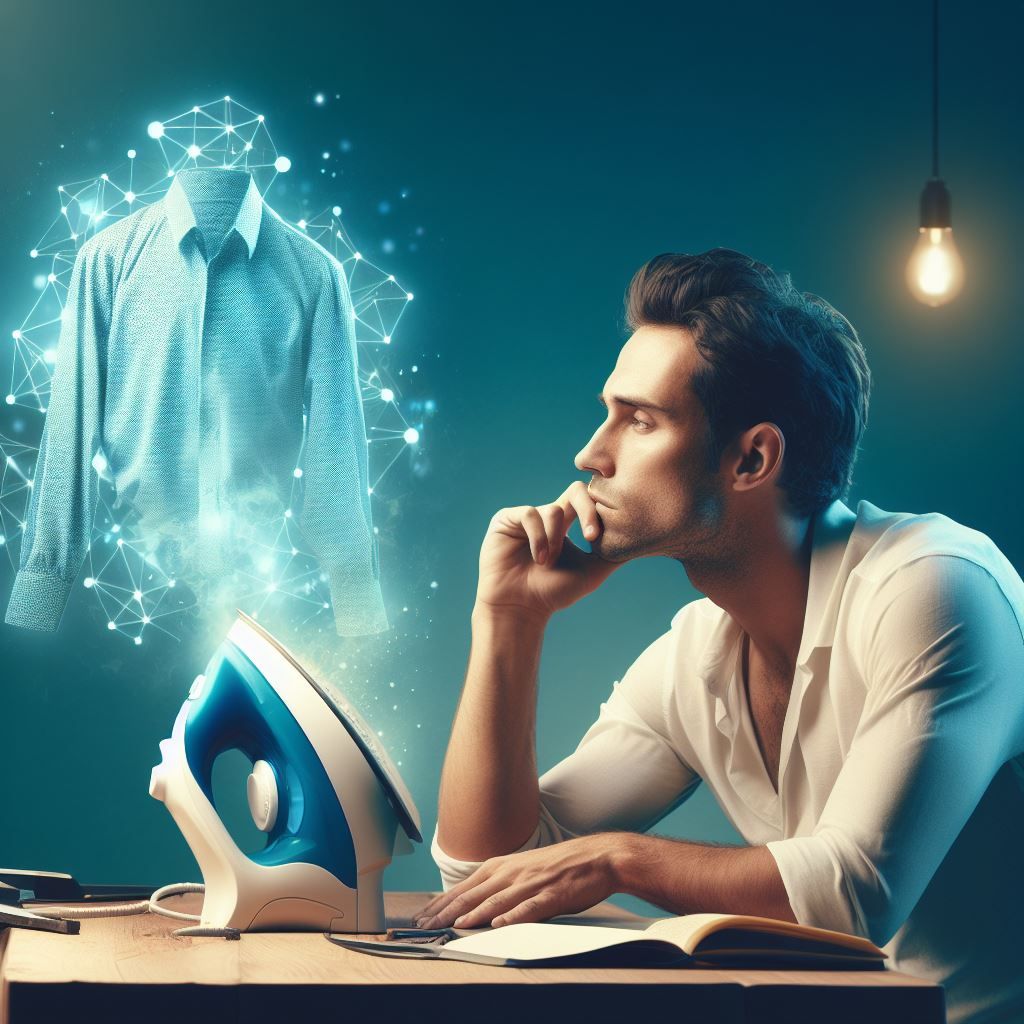
What are some real-world applications of generative AI in creative fields?
Generative AI has found applications across various domains that involve creativity, such as art, music, literature, design, engineering, science, medicine, business, education, entertainment, and more. Here are some examples of how generative AI is being used in these fields:
Art
Generative AI has enabled machines to create stunning artwork, illustrations, and even 3D designs that mimic or surpass human artistic abilities. Artists and designers often use these AI-generated pieces as a starting point for their creative projects or as a source of inspiration.
One of the most famous examples of generative AI in art is the Portrait of Edmond Belamy, a painting created by a GAN that was sold for $432,500 at Christie’s auction house in 2018. The painting was part of a series of portraits generated by a collective of French artists called Obvious, who used a GAN to produce images of fictional members of a fictional family called the Belamy.
Another example of generative AI in art is Artbreeder, an online platform that allows users to create and explore images using GANs. Users can upload their own images or choose from a gallery of images and then modify them using various parameters such as style, color, mood, and complexity. Users can also mix and blend different images to create new ones. Artbreeder has been used by artists, designers, game developers, and hobbyists to create unique and diverse artworks.
Music
Generative AI has enabled machines to create music in a variety of genres and styles that resemble or challenge human musical compositions. Musicians and composers often use these AI-generated pieces as a basis for their own musical creations or as a way to experiment with new sounds and melodies.
One of the most prominent examples of generative AI in music is AIVA, an AI composer that creates original music for various purposes such as films, games, commercials, and podcasts. AIVA uses deep learning to analyze thousands of musical pieces from different genres and eras and then generates new music based on the user’s preferences and emotions. AIVA has been recognized by the Society of Composers & Lyricists (SCL) as an official composer member.
Another example of generative AI in music is Jukebox, an AI system that generates music with lyrics in the style of various artists such as Frank Sinatra, Elvis Presley, Katy Perry, and Metallica. Jukebox uses neural networks to model raw audio waveforms from songs and then generates new songs based on the user’s input. Jukebox can also remix existing songs or create mashups of different songs.
Literature
Generative AI has enabled machines to create text in various forms such as stories, poems, essays, articles, reviews, and more that emulate or challenge human literary expression. Writers and readers often use these AI-generated texts as a source of inspiration, entertainment, or education.
One of the most impressive examples of generative AI in literature is GPT-3, a language model that can produce human-like text on any topic given a few words or sentences as input. GPT-3 uses deep learning to analyze billions of words from the internet and then generates new text based on the user’s prompt. GPT-3 has been used to create various types of texts such as stories, poems, essays, articles, reviews, and more. Some examples of GPT-3 generated texts are:
- A short story about a lion and a mouse that became friends
- A poem about love in the style of Shakespeare
- An essay on why homework should be banned
- An article on how to cope with stress during the pandemic
- A review of the latest iPhone model
Another example of generative AI in literature is Replika, an AI chatbot that can create personalized stories for users based on their preferences and emotions. Replika uses natural language processing and deep learning to understand the user’s input and then generates a story that matches the user’s mood, genre, and characters. Replika can also engage in conversations with the user and provide emotional support.
Design
Generative AI has enabled machines to create design solutions for various purposes such as logos, websites, products, and more that meet or exceed human design standards. Designers and clients often use these AI-generated solutions as a reference or a prototype for their own design projects or as a way to save time and money.
One of the most popular examples of generative AI in design is Logojoy, an online platform that allows users to create professional logos in minutes using GANs. Users can choose from a variety of styles, colors, fonts, icons, and layouts and then customize them according to their preferences. Logojoy also provides feedback and suggestions to help users improve their logos.
Another example of generative AI in design is Wix ADI, an AI system that creates personalized websites for users based on their needs and goals. Wix ADI uses natural language processing and deep learning to understand the user’s input and then generates a website that matches the user’s industry, style, and features. Wix ADI also allows users to edit and modify their websites as they wish.
Engineering
Generative AI has enabled machines to create engineering solutions for various challenges such as structures, circuits, materials, and more that optimize or surpass human engineering performance. Engineers and researchers often use these AI-generated solutions as a guide or a benchmark for their own engineering projects or as a way to discover new possibilities and innovations.
One of the most impressive examples of generative AI in engineering is NASA’s antenna design, which was created by an evolutionary algorithm that mimics natural selection. The algorithm generated thousands of antenna designs and then selected the best ones based on their fitness criteria such as size, weight, power consumption, and signal quality. The algorithm produced an antenna design that was superior to any human-designed antenna in terms of efficiency and performance.
Another example of generative AI in engineering is Autodesk’s generative design, which is a software tool that helps engineers create optimal design solutions for various problems such as structures, vehicles, products, and more. The software tool uses cloud computing and machine learning to generate thousands of design alternatives based on the user’s specifications and constraints. The software tool also evaluates and compares the design alternatives based on various criteria such as cost, material, weight, strength, and environmental impact.
Science
Generative AI has enabled machines to create scientific solutions for various questions such as molecules, proteins, drugs, and more that advance or revolutionize human scientific knowledge. Scientists and researchers often use these AI-generated solutions as a source of inspiration or validation for their own scientific projects or as a way to accelerate scientific discovery and innovation.
One of the most remarkable examples of generative AI in science is DeepMind’s AlphaFold, an AI system that predicts the 3D structure of proteins from their amino acid sequences. Proteins are essential molecules that perform various functions in living organisms such as catalyzing reactions, transporting substances, fighting diseases, and more. The 3D structure of proteins determines their function and behavior. However, predicting the 3D structure of proteins from their amino acid sequences is a very difficult and time-consuming task that requires expensive experiments or complex simulations. AlphaFold uses deep learning to analyze millions of protein structures from public databases and then predicts the 3D structure of new proteins with high accuracy and speed. AlphaFold has been recognized as a breakthrough in computational biology that could have profound implications for medicine, biotechnology, agriculture, and more.
Another example of generative AI in science is IBM’s RXN for Chemistry, an AI system that predicts the outcomes of chemical reactions from their reactants and their conditions. Chemical reactions are the processes that transform one or more substances into new substances with different properties and characteristics. However, predicting the outcomes of chemical reactions from their reactants and conditions is a very challenging and tedious task that requires extensive knowledge and experience in chemistry. RXN for Chemistry uses deep learning to analyze millions of chemical reactions from public databases and then predicts the outcomes of new chemical reactions with high accuracy and speed. RXN for Chemistry has been hailed as a powerful tool for synthetic chemistry that could facilitate the development of new drugs, materials, and products.
Medicine
Generative AI has enabled machines to create medical solutions for various problems such as diagnosis, treatment, prevention, and more that improve or save human health and lives. Doctors and patients often use these AI-generated solutions as a support or a complement for their own medical decisions or actions or as a way to enhance medical quality and efficiency.
One of the most impressive examples of generative AI in medicine is Insilico Medicine’s drug discovery, which was created by a GAN that designs new molecules for various diseases. Drugs are substances that can alter the function or structure of living cells or organisms. However, discovering new drugs for specific diseases is a very costly and time-consuming process that involves many trials and errors. Insilico Medicine uses a GAN to generate novel molecules that target specific proteins or pathways related to certain diseases. The GAN also predicts the properties, toxicity, and efficacy of the generated molecules. Insilico Medicine has been able to discover new drugs for various diseases such as cancer, Alzheimer’s, Parkinson’s, and more in a matter of days or weeks.
Another example of generative AI in medicine is Babylon Health’s symptom checker, which is an AI system that diagnoses medical conditions from the user’s symptoms and history. Symptoms are signs or indicators that something is wrong with the body or mind. However, diagnosing medical conditions from symptoms can be very difficult and complex, especially for non-experts or people who lack access to health care. Babylon Health uses natural language processing and deep learning to understand the user’s input and then generates a list of possible medical conditions that match the user’s symptoms and history. Babylon Health also provides advice on what to do next, such as self-care, consultation, or emergency.
Business
Generative AI has enabled machines to create business solutions for various goals such as marketing, sales, customer service, and more that increase or optimize human business performance and value. Business owners and managers often use these AI-generated solutions as a strategy or a tactic for their own business operations or as a way to gain competitive advantage and customer satisfaction.
One of the most popular examples of generative AI in business is Phrasee, an AI system that creates catchy and effective email subject lines, headlines, slogans, and more for various marketing campaigns. Marketing is the process of promoting and selling products or services to potential customers. However, creating marketing content that attracts attention and persuades action can be very challenging and subjective. Phrasee uses natural language processing and deep learning to analyze millions of marketing content from different industries and domains and then generates new content that matches the user’s brand voice, tone, and message. Phrasee also measures the performance of the generated content based on various metrics such as open rate, click rate, conversion rate, and revenue.
Another example of generative AI in business is Copy.ai, an online platform that helps users create various types of content for their businesses such as logos, websites, social media posts, blogs, newsletters, ads, and more using GANs. Users can enter their business name, description, keywords, or images and then choose from a variety of templates and styles. Copy.ai then generates content that suits the user’s needs and preferences. Copy.ai also allows users to edit and customize their content as they wish.
Education
Generative AI has enabled machines to create educational solutions for various purposes such as learning, teaching, testing, and more that enhance or support human educational outcomes and experiences. Learners and educators often use these AI-generated solutions as a resource or a tool for their own educational activities or as a way to improve educational quality and accessibility.
One of the most innovative examples of generative AI in education is Quizlet’s Diagrams, an AI system that creates interactive diagrams for various subjects such as biology, geography, history, and more. Diagrams are visual representations of information that help learners understand and remember concepts and relationships. However, creating diagrams can be very tedious and time-consuming, especially for complex or dynamic topics. Quizlet uses computer vision and deep learning to analyze images of various topics from public sources and then generates diagrams that label and explain the key elements and features of the images. Quizlet also provides quizzes and games based on the diagrams to help learners test and reinforce their knowledge.
Another example of generative AI in education is Duolingo’s Stories, an AI system that creates engaging and personalized stories for language learners. Stories are narratives that convey meaning and emotion through characters, events, and dialogue. Stories are also effective tools for language learning, as they expose learners to authentic and contextualized language use and culture. However, creating stories that match the learner’s level, interest, and goal can be very difficult and subjective. Duolingo uses natural language processing and deep learning to analyze millions of stories from different languages and genres and then generates new stories that adapt to the learner’s proficiency, preference, and progress. Duolingo also provides feedback and exercises based on the stories to help learners improve their vocabulary, grammar, pronunciation, and comprehension.
Conclusion
Generative AI is a powerful and promising technology that can augment human creativity in various ways. Generative AI can help humans explore new possibilities, generate novel ideas, overcome creative blocks, find new sources of inspiration, solve complex problems, optimize solutions, collaborate with other humans and machines, and more. Generative AI can also create content across various domains such as art, music, literature, design, engineering, science, medicine, business, education, entertainment, and more.
However, generative AI also poses some challenges and opportunities for the future of creativity. Some of the challenges include:
- The ethical and social implications of generative AI for human creativity. How can we ensure that generative AI is used for good and not for evil? How can we protect the rights and responsibilities of human creators and consumers of generative AI content? How can we prevent or detect the misuse or abuse of generative AI content such as plagiarism, fraud, deception, manipulation, or harm?
- The technical and practical limitations of generative AI for human creativity. How can we improve the quality and reliability of generative AI content? How can we evaluate and measure the creativity of generative AI content? How can we integrate and interface generative AI with human creativity? How can we balance the trade-off between human control and machine autonomy in generative AI?
- The psychological and cultural effects of generative AI for human creativity. How does generative AI affect our perception and appreciation of human creativity? How does generative AI influence our motivation and confidence in our own creativity? How does generative AI impact our identity and expression as creative beings? How does generative AI shape our values and norms as creative societies?
Some of the opportunities include:
- The educational and developmental benefits of generative AI for human creativity. How can we use generative AI to enhance our creative skills and abilities? How can we use generative AI to inspire our creative passions and interests? How can we use generative AI to foster our creative growth and potential?
- The collaborative and co-creative possibilities of generative AI for human creativity. How can we use generative AI to collaborate with other humans in creative processes? How can we use generative AI to co-create with machines in creative processes? How can we use generative AI to create new forms of creativity that transcend human or machine capabilities?
- The innovative and transformative outcomes of generative AI for human creativity. How can we use generative AI to create new solutions for existing problems or new problems for existing solutions? How can we use generative AI to create new products or services that add value or delight to our lives? How can we use generative AI to create new experiences or stories that enrich or enlighten our minds?
Generative AI is not a threat or a replacement for human creativity. It is a catalyst and a complement for human creativity. Generative AI is not a competitor or a rival for human creativity. It is a partner and a friend for human creativity. Generative AI is not an end or a goal for human creativity. It is a means and a tool for human creativity.
We invite you to join us in exploring the fascinating world of generative AI and its implications for human creativity. We hope you enjoyed this article and learned something new or interesting. We also encourage you to share your thoughts and opinions on this topic with us or with others. We look forward to hearing from you.
Thank you for reading.
Note: In line with my passion to use technology to help humans’s efficiency and creativity, this article has been written with the support of BingAI & Bing Image Creator.
References:
https://hbr.org/2023/07/how-generative-ai-can-augment-human-creativity
https://www.solulab.com/generative-ai-with-human-creativity/
https://www.folio3.ai/blog/generative-ai-can-augment-human-creativity/
https://venturebeat.com/business/why-we-need-augmented-creativity-to-reignite-growth-in-2021/
https://www.weforum.org/agenda/2023/02/ai-can-catalyze-and-inhibit-your-creativity-here-is-how/
https://link.springer.com/chapter/10.1007/978-3-030-78462-1_13